Mitral regurgitation (MR) is one of the most common valvular disorders, affecting about one out of 15 older adults — and it’s also one of the most challenging to assess with artificial intelligence (AI). Timothy J. Poterucha, MD, a cardiologist at NewYork-Presbyterian and Columbia and director of Innovation in the Columbia University Echocardiography Laboratory, believes that interpretation of echocardiography to detect MR can be potentially improved with the help of deep learning (DL), a type of AI analysis.
Dr. Poterucha is one of three principal investigators with the CRADLE (Cardiovascular and Radiologic Deep Learning Environment) Lab, a NewYork-Presbyterian research collaborative that brings together expert cardiologists and data scientists from Columbia and Weill Cornell Medicine. Their goal is to build AI algorithms and models aimed at improving cardiac care, and he recently led a first-of-its-kind study to develop and validate a novel DL system to detect MR on echocardiograms. The results were published in Circulation in June 2024. Below, he provides background on the study, what the researchers found, and what the results mean for future treatment of MR.
Opportunities and Challenges
Deep learning is particularly useful for looking at complex data and we believe that it will help us better analyze imaging data and diagnostic tests. We chose to develop an AI model for mitral regurgitation because it's clinically important and exceptionally challenging. That combination gave us the perfect opportunity to use our laboratory, which houses an enormous amount of data on NewYork-Presbyterian patients at Weill Cornell Medicine and Columbia, to develop and test this model.
Teaching an AI model to do what a human cardiologist can do is complex. In our study, we had to teach it to recognize MR on imaging, to identify which echocardiogram clips deal with the mitral valve and involve MR, and to determine how much MR is present. We also had to teach it to integrate the individual video-level predictions into the final study diagnosis. It was an arduous process but ultimately, we think computer-assisted tools will help us improve our overall accuracy and reduce potential mistakes.
Research Goals and Methods
For this particular research, our main goal was to build an AI model that could assess the presence and severity of MR at an academic echocardiography lab level; validate these findings in multiple hospital settings; and look at the ability of the model to identify potential misdiagnoses, misclassifications, or mistakes, with the idea that it could also be used as a quality improvement tool.
For the study, we developed the DELINEATE-MR system, an AI-based approach that processes complete echocardiogram studies to assess MR severity on a four-step scale: none/trace, mild, moderate, and severe. We used human cardiologist readings as the reference standard.
An internal data set was comprised of patients undergoing a complete echocardiogram at multiple NewYork-Presbyterian and Columbia locations between 2015 and 2023. An external test set was made up of patients undergoing complete echocardiograms at NewYork-Presbyterian and Weill Cornell Medicine from 2019 to 2021. Some 61,689 echocardiograms were used for training, validation, and internal testing, with an additional 8,208 for external testing.
Key Findings
Our analysis showed that the DELINEATE-MR model demonstrated high accuracy: 82% exact agreement with cardiologists and AUROC of 0.98 or the detection of moderate or severe MR. In the external validation, the system achieved 79% accuracy.
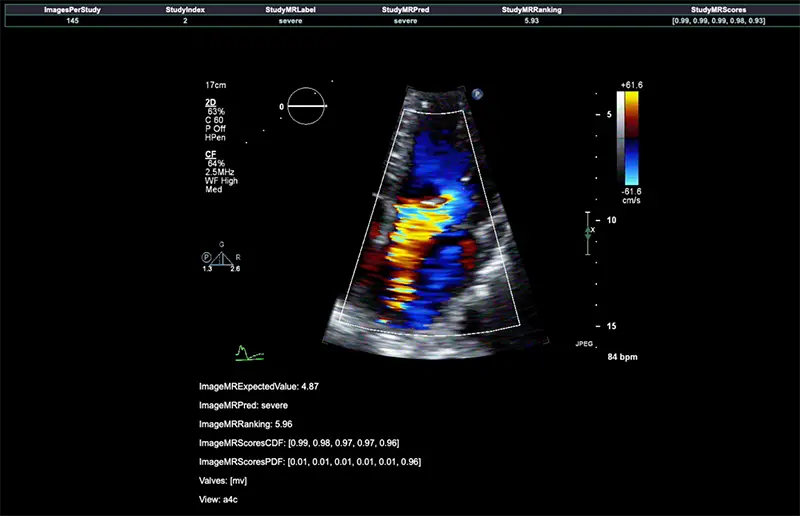
In this echocardiogram, severe mitral regurgitation is shown as a bright jet of color. The DELINEATE-MR system can correctly identify the presence of severe mitral regurgitation. It is hoped that AI tools like this one may help cardiologists more accurately and efficiently diagnose heart disease.
We trained our model using cardiologist assessments and demonstrated substantial agreement with clinical interpretations in the internal and external test sets. Notably, most misclassification disagreements were between none/trace and mild MR. Accuracy was slightly higher using multiple echocardiogram views (82%) compared to just the apical 4-chamber view (80%).
The model's performance was robust across different MR types, showing high accuracy in classifying both primary and secondary MR. However, it did show slightly lower performance in eccentric MR cases.
When you have the right approach, the right team, and the right kind of data, you can develop AI models that can match or even exceed human experts. And that’s what ours did. We were able to bring together data from echocardiograms from tens of thousands of patients and use the latest techniques in computer science and AI to analyze it, meshed with the opinion of a dozen cardiologists.
Looking to the Future
Our study is the first time an AI model has been used to assess valve regurgitation of any kind. Our next step is to take this process and apply it to every important finding on an echocardiogram and the diseases that affect the other three heart valves, and then study how these models can be integrated inside of clinical practice to show that it can make a difference in patient care.
These AI models are hungry for data. We need large-scale data to approach these problems, and we need it to be as diverse as possible. NewYork-Presbyterian is the perfect place to do this work because we can build models on very large scales of data coming from multiple hospitals in the most diverse patient population imaginable, New York City.
It's equally important to note that these tools are not meant to replace physicians. Even if we can teach an AI model to look at an individual video better than a cardiologist can, the cardiologist still has the advantage of knowing the patient extremely well. They know the subtleties of their symptoms and how their symptoms have changed over the context of months or years. That insight is irreplaceable. We see these tools as helpers – they’re going to help us do a better job taking care of patients by allowing us to spend less time looking at images and more time being with and taking care of people.